Recent Breakthroughs in AI and Healthcare; March Roundup
- Shafi Ahmed
- Apr 7
- 8 min read
Updated: May 5
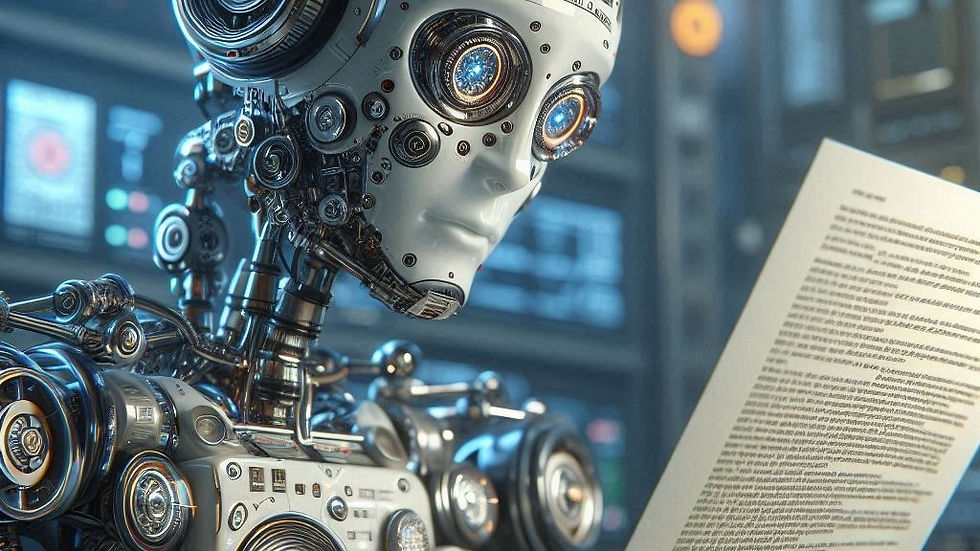
By Professor Shafi Ahmed
Surgeon | Futurist | Innovator | Entrepreneur | Humanitarian | International Keynote Speaker
As AI transforms healthcare, it challenges us to rethink the very fabric of medicine—not as a static practice, but as a dynamic fusion of human expertise and artificial intelligence, pushing boundaries to create a future where precision and accessibility are no longer aspirations but realities.”
Artificial Intelligence (AI) continues revolutionizing healthcare, offering innovative solutions that enhance diagnostic accuracy, personalize treatment plans, and improve patient outcomes. In this edition of AI Horizons, I will explore the latest research papers published on AI in healthcare, highlighting significant advancements and their implications. I will also delve into the recently viral Ghibli AI trend and its potential impact in healthcare. Recent Research Breakthroughs in AI and Healthcare
AI at the Frontier of Modern Medicine:
In the comprehensive review Artificial Intelligence for Medicine 2025: Navigating the Endless Frontier, researchers delve into how AI transforms healthcare through enhanced diagnostics, precision treatments, and drug discovery. By integrating diverse data types—clinical, imaging, physiological, and omics data—AI enhances precision in understanding disease mechanisms, predicting outcomes, and tailoring treatments.
Significant advancements include AI's ability to analyze phenotypic data, enabling early detection of conditions like dementia and polycystic ovary syndrome (PCOS). Innovations in surgical robotics, supported by AI, are advancing from assistive tools to autonomous systems, exemplified by the Levels of Autonomy in Surgical Robotics (LASR) classification system. Meanwhile, breakthroughs in drug development utilize AI to predict protein and RNA structures, streamlining candidate discovery and optimizing experimental processes. However, challenges persist, including computational demands, ethical concerns, and the complexity of interpreting multimodal datasets.
The paper highlights how AI addresses societal issues, such as aging populations and declining birth rates, through novel technologies in reproductive health, cognitive monitoring, and smart environments for elder care. While these advances underscore AI's promise of reshaping medicine, the authors stress the need for standardization, ethical guidelines, and collaboration between AI experts and clinicians to realize its full potential.
Redefining Patient Stratification: Not All Risks Are Equal
A pivotal study in JAMIA questions conventional disease risk models. Instead of merely predicting disease onset, researchers propose integrating the severity of downstream outcomes—a crucial but overlooked component. Using sepsis as a case study, the authors highlight a critical gap in conventional predictive models, which often overlook how diseases impact outcomes like mortality.
The study analyzes ICU data from Michigan Medicine (2016–2020) and Beth Israel Deaconess Medical Center (2008–2012). Results indicate a weak correlation between the risk of sepsis onset and its effect on mortality (Spearman coefficients of 0.35 and 0.31). This disconnect implies that patients at high risk of developing sepsis are not necessarily those most vulnerable to severe outcomes, such as death. Notably, older patients—more susceptible to severe sepsis outcomes—are often missed under traditional stratification models.
The authors propose a combined predictive approach integrating sepsis risk with its impact on mortality. This methodology identifies different patient populations compared to risk-based models alone, ensuring more equitable resource allocation in clinical settings. While sepsis serves as a proof-of-concept, the approach has broader implications for conditions like COVID-19, where disease severity significantly influences treatment outcomes. The study urges a paradigm shift in AI-driven healthcare to align predictive tools with patient-centered goals better.
Understanding the “Biology” of Language Models
The paper "Biology of a Large Language Model" demystifies the internal workings of Anthropic's Claude 3.5 Haiku. Using advanced tools, like attribution graphs and a novel circuit tracing methodology, the researchers reverse-engineer how these models process information internally. Using "replacement models," they identify human-interpretable features as computational units akin to cells in biology and trace connections between them.
Key findings include the model's ability to perform multi-step reasoning, plan responses ahead, and generalize computations across languages and contexts. For example, Claude 3.5 Haiku plans rhymes in poetry and identifies medical diagnoses based on symptoms, showcasing abstract reasoning and problem-solving. The research also uncovers mechanisms behind phenomena like entity recognition, refusal of harmful requests, and hallucinations.
Findings revealed Claude’s capabilities in abstract reasoning—identifying medical diagnoses, generating rhymes, and avoiding harmful outputs. While only partial transparency was achieved, this research sets a precedent for interpretable AI, essential for safe medical applications.
AI-Powered Therapy: Therabot Delivers Clinical-Grade Mental Health Support
In a randomized controlled trial published in NEJM AI, Therabot, a generative AI chatbot, was evaluated for its effectiveness in treating major depressive disorder (MDD), generalized anxiety disorder (GAD), and high-risk feeding and eating disorders (CHR-FED). For instance, MDD symptoms decreased by an average of −6.13 at four weeks, while CHR-FED reductions averaged −9.83. Therapeutic alliance ratings for Therabot matched those of human therapists, and participants spent over six hours actively engaging with the chatbot.
These findings underscore Therabot's potential to deliver scalable, personalized mental health care that maintains clinical efficacy. While promising, the study highlights the need for further validation with larger sample sizes to confirm effectiveness and generalizability. This research advances AI integration in mental health, addressing accessibility challenges in evidence-based interventions for diverse patient populations.
Your Voice, Your Diagnosis: AI Detects Depression Through Speech
The Annals of Family Medicine evaluated Kintsugi Voice, an AI voice biomarker tool, on 14,898 individuals. With just 25 seconds of speech, it detected moderate to severe depression with 71.3% sensitivity and 73.5% specificity.
The findings highlight the potential of machine learning in augmenting depression screening in primary care, addressing the current gap where less than 4% of patients are screened. The study underscores this technology's non-invasive, scalable nature, which could integrate seamlessly into virtual consultations. While promising, the authors call for further research to refine the tool, address demographic variability, and validate its clinical deployment.
AI Against the Next Pandemic
A study from the University of Oxford explores how AI can revolutionize pandemic preparedness, and enhance genetic data analysis for vaccine development. By combining population and individual-level data, including from wearables, AI can predict outbreak patterns, vaccine targets, and pathogen evolution, like SARS-CoV-2. While AI offers transformative possibilities, the authors stress the importance of collaboration across academia, industry, and policy organizations to ensure ethical deployment and robust evaluation of AI models. They caution against relying solely on AI, advocating for human feedback integration to address limitations like data quality and accessibility. This research underscores AI's role in advancing global health, particularly in resource-limited settings, and calls for transparent, accountable practices to maximize its impact on pandemic response systems.
Faster, Smarter, Safer: AI in Fetal Ultrasound Scanning
In a randomized controlled trial in NEJM AI, AI-assisted fetal anomaly ultrasound scans significantly boosted sensitivity (88.9%) and specificity (98.0%) while reducing scan time (11.4 vs. 19.7 minutes). Pregnant participants (with and without congenital heart disease in fetuses) and sonographers were randomized to perform scans using either AI assistance or standard methods. The AI tool helped identify and save 13 image planes and measure four biometrics, though it did not automate congenital heart disease diagnosis.
The study evaluates the impact of artificial intelligence (AI) in fetal anomaly ultrasound scanning through a randomized, single-center trial conducted in a teaching hospital. Sonographer cognitive load was lower in the AI group, and AI-assisted measurements demonstrated superior repeatability and reproducibility. The findings suggest that AI can enhance diagnostic accuracy, efficiency, and clinician workload in routine fetal ultrasound scans without compromising safety.
Diagnosing Celiac Disease with Pathologist-Level AI
A NEJM AI study introduced a machine learning model for celiac disease diagnosis using duodenal biopsy images. CD diagnosis often relies on histological evaluation, which suffers from interpathologist agreement rates of 80% or less. This AI model, trained on 3,383 whole-slide images from four hospitals, was evaluated on an independent test set of 644 previously unseen scans. It achieved over 95% accuracy, sensitivity, and specificity, with an area under the receiver operating characteristic curve exceeding 99%. The AI’s performance matched that of specialist pathologists in interobserver agreement.
The model’s success highlights its potential to reduce diagnostic variability and expedite workflows in histopathology. This groundbreaking approach demonstrates the transformative role of AI in enhancing diagnostic precision and efficiency, addressing critical gaps in healthcare delivery.
Emergency Rooms Get Smarter with AI Triage Support
A study titled "Impact of Artificial Intelligence–Based Triage Decision Support on Emergency Department Care” examines the impact of an AI-based clinical decision support (CDS) tool on emergency department (ED) triage. Conducted across three sites with 174,648 ED visits, the AI-informed triage system improved triage performance, identifying high-acuity patients with critical care needs more accurately (78.8% to 83.1%, P<0.001). Post-implementation, low-acuity visits increased, optimizing patient distribution. Median time to initial care area decreased by 33.3%, while time to ED disposition and departure improved. However, variability in nurses’ agreement with AI recommendations highlighted gaps in consistency.
Streamlining Patient Messaging with NLP Models
An NLP-based AI system for routing outpatient patient portal messages showed impressive results: response times dropped by 1 hour, resolution times by 22.5 hours, and staff workload reduced. Achieving over 97% accuracy, this model highlights the potential of AI to streamline communication, reduce clinician workload, and enhance efficiency in healthcare settings, addressing challenges like provider burnout and delayed patient interactions.
EchoNet-Liver: Echocardiograms Reveal Hidden Liver Disease
A study published in NEJM AI introduces EchoNet-Liver, a deep-learning pipeline designed for opportunistic chronic liver disease (CLD) screening using echocardiography. Using cardiac imaging, echoNet-Liver, developed using 1.5 million echo videos, detects cirrhosis (AUROC 0.830) and steatotic liver disease (AUROC 0.769). By identifying high-quality subcostal images, EchoNet-Liver enables early detection of asymptomatic CLD, which affects over 1.5 billion adults globally. This approach highlights the potential of AI-enhanced imaging to improve diagnostic workflows and patient outcomes, offering a scalable solution for addressing undiagnosed liver diseases.
Accountability in the Age of AI Medicine
A paper published in PMC critically addresses AI's ethical and safety challenges in clinical decision-making. As AI systems increasingly assist in critical decision-making, traditional accountability frameworks—relying on clinicians' control and understanding—face limitations. Using an AI system developed for sepsis treatment as a case study; the authors highlight the gaps in moral responsibility when clinicians have limited control over or understanding of AI-driven recommendations.
The paper emphasizes the need to update safety assurance from static models to dynamic ones, adapting to real-world deployment and evolving clinical contexts. It calls for a collaborative approach involving AI developers and safety engineers to address risks like unintended consequences, opaque decision-making, and epistemic uncertainties. This work underscores the ethical and operational challenges of integrating AI into safety-critical healthcare environments.
The Ghibli Effect AI Trend: A New Frontier for AI in Healthcare
The "Ghibli Effect" AI trend, which transforms real-world images into the whimsical, hand-drawn style of Studio Ghibli animations, has sparked both fascination and debate. Tools like ChatGPT’s AI image generator enable users to reimagine photos as dreamlike scenes reminiscent of films like "Spirited Away."
In healthcare, the Ghibli Effect’s aesthetic could humanize technology. For instance, AI-generated visuals might simplify medical concepts for patients, easing anxiety through familiar, comforting imagery. Predictive analytics and automated workflows, likened to a "Ghibli world," could enhance diagnostics and reduce clinician burnout by streamlining tasks.
However, ethical concerns persist. Over-reliance on stylized AI outputs might obscure critical health data or create unrealistic patient expectations. Additionally, the trend’s detachment from Studio Ghibli’s artisanal ethos—rooted in human storytelling—mirrors broader fears about AI replacing creativity and empathy in healthcare. Data privacy risks and potential biases in AI training data further complicate its adoption. While the Ghibli Effect showcases AI’s potential to blend art and utility, balancing innovation with ethical rigor remains essential to avoid diminishing the human element in both art and medicine.
Final Thoughts
The integration of AI into healthcare is yielding transformative results, from accelerating disease diagnosis to enhancing patient care and clinician well-being. Recent research underscores the potential of AI to revolutionize various facets of healthcare, offering promising solutions to longstanding challenges. However, robust regulatory frameworks and ethical safeguards are essential to mitigate risks as AI becomes increasingly embedded in clinical workflows.
AI is shaping the future of healthcare, but its success depends on responsible innovation, cross-disciplinary collaboration, and global regulatory harmonisation.
Comments